The Real Costs of
Fine-Tuning LLMs
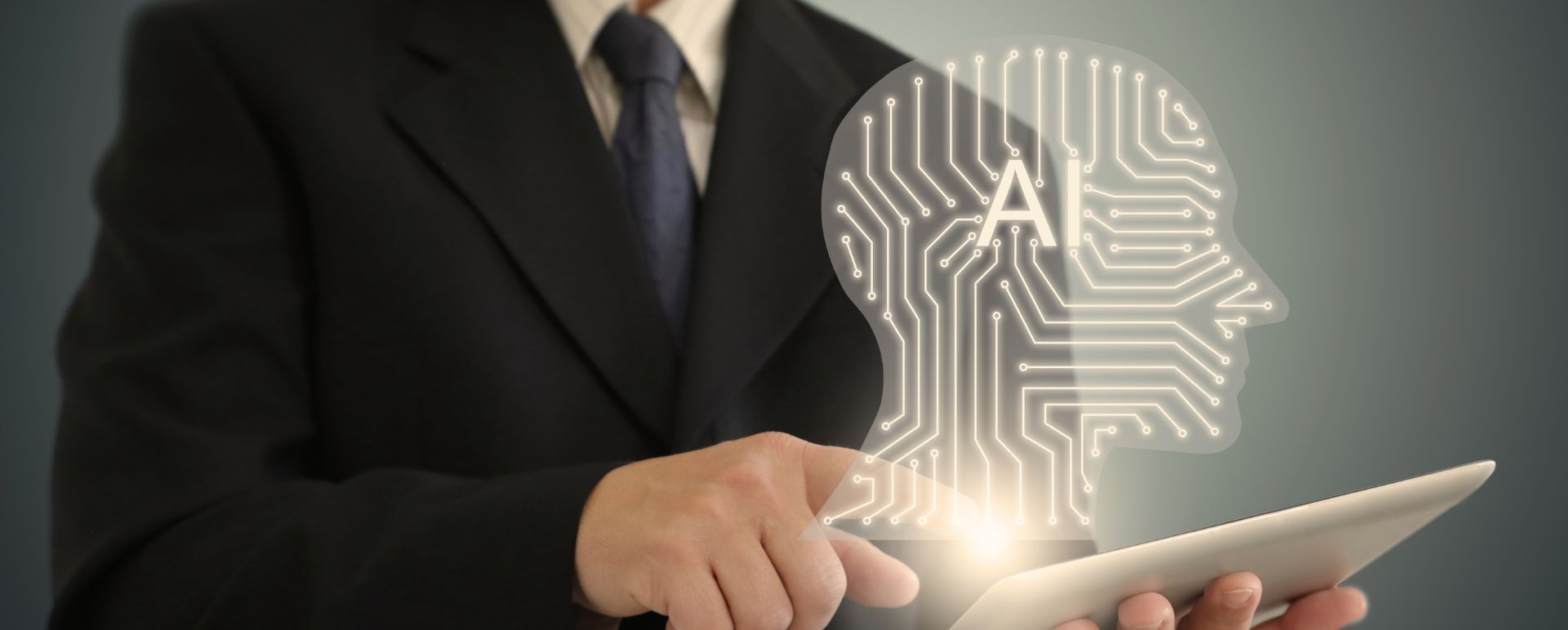
11th Aug 2024
When You Should and Shouldn't Train Your Own Models?
Large Language Models (LLMs) have become the backbone of AI-powered applications. From chatbots to code assistants, enterprises are investing millions into fine-tuning these models to meet specific needs. But here’s the problem—fine-tuning is expensive, rarely necessary, and often a trap.
People assume that fine-tuning will make an LLM “understand” their business better, generate more accurate responses, or improve efficiency. In reality, fine-tuning is often overkill. Most businesses don’t need it, and those that do often do it wrong. The cost, both financial and technical, can be staggering. So, when should you fine-tune an LLM? And when should you just use a better prompting strategy or retrieval-augmented generation (RAG)?
Let’s break it down.
1. Compute and Infrastructure Costs
- Fine-tuning requires hundreds of GPUs or TPUs, and unless you have an in-house high-performance cluster, cloud costs will bleed your budget dry.
- Even modest fine-tuning on a model like Llama 3-70B can cost upwards of $50,000 per training run.
- If you’re using FP16 precision, expect double the memory footprint compared to INT8 quantization.
2. Data Preparation Nightmares
- High-quality, domain-specific data is mandatory, and most businesses simply don’t have enough labeled data to make fine-tuning worthwhile.
- Cleaning data takes months—mislabeling can make your model worse than the base model.
- Data drift is real. Your fine-tuned model will decay fast if the industry changes or new knowledge emerges.
3. Model Maintenance and Degradation
- Once fine-tuned, your model is no longer in sync with base model updates. If OpenAI releases a better GPT version, your fine-tuned version lags behind.
- Fine-tuning a general-purpose model locks you in—you now own the burden of monitoring, updating, and debugging forever.
4. Inferencing Costs Skyrocket
- Fine-tuned models can be 2-5x more expensive to run than off-the-shelf models.
- Running a dedicated inference server for fine-tuned models is not cheap, especially for low-latency applications.
When Should You Fine-Tune?
Fine-tuning is justified only in a few scenarios:
- You need an LLM with industry-specific knowledge that is absent from public models (e.g., a medical diagnostic AI trained on proprietary datasets).
- You require strict control over the output format (e.g., legal contracts, compliance documents where hallucinations are unacceptable).
- Your task involves highly specialized jargon or non-standard language usage (e.g., protein-folding research, financial risk modeling).
- You need a model to "imitate" specific behavior, tone, or style at scale (e.g., a chatbot trained to sound like a brand's founder).
When Shouldn’t You Fine-Tune? (AKA, 90% of Cases)
- Your goal is just to improve response accuracy. RAG (Retrieval-Augmented Generation) is a better option. Instead of modifying the model itself, just give it access to better information.
- You think fine-tuning will "fix" hallucinations. It won’t. Hallucinations come from architectural limitations, not lack of fine-tuning.
- You want the model to “understand your business better.” Just feed it structured context in your prompts.
- You think fine-tuning will improve reasoning ability. It won’t. Fine-tuning affects knowledge, not reasoning. If you want better reasoning, use structured prompt engineering or chain-of-thought methods.
Fine-tuning a large LLM like GPT-3 can cost anywhere from $80,000 to over $1 million, depending on dataset size and compute needs
Fine-tuning is a trap for most businesses. It’s expensive, complex, and usually unnecessary. Before even considering it, try RAG, better prompting, and lighter tuning methods like LoRA. If your use case still demands fine-tuning, be prepared for a long-term commitment. Otherwise, you're just burning cash for marginal gains.
Share this post
Have a feedback?
Let's discuss more about it! Just write your feedback below and I'll reach out to you soon